引用本文: | 陈 帼,徐新刚※,杜晓初,杨贵军,赵晓庆,魏鹏飞,王玉龙,范玲玲.基于PLS 和组合预测方法的冬小麦收获
指数高光谱估测[J].中国农业信息,2019,31(2):28-38 |
| |
|
本文已被:浏览 1799次 下载 652次 |
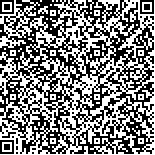 码上扫一扫! |
|
基于PLS 和组合预测方法的冬小麦收获
指数高光谱估测 |
陈 帼1,2,3, 徐新刚※4,3, 杜晓初1, 杨贵军4,3, 赵晓庆4,3, 魏鹏飞1,2,3, 王玉龙4,3, 范玲玲4,3
|
1.湖北大学资源与环境学院,武汉430062;2.农业部农业遥感机理与定量遥感重点实验室/ 北京农业信息技术研究中心,北京100097;3.国家农业信息化工程技术研究中心,北京100097;4.农业部农业遥感机理与定量遥感重点实验室/ 北京农业信息
技术研究中心,北京100097
|
|
摘要: |
【目的】通过遥感反演测量收获指数(HI),可节省时间和人力,但需要提高精度。
通过权重最优组合算法改善收获指数估算精度,为基于多时相多光谱信息的HI 遥感估算提
供新方法参考。【方法】利用测定的冬小麦多个关键生育期的冠层光谱数据,对筛选的44 种
常用植被指数与实测收获指数进行相关性分析,挑选出每个育期中5 种最优的典型植被指
数;应用偏最小二乘(PLS)的方法建模,分别得到基于单个生育期光谱信息的HI 遥感估测
模型;借鉴组合预测原理,应用组合预测方法对全部单生育期的各HI 光谱模型赋予最优权
重,最终构建基于多生育期数据的HI 光谱组合预测模型。【结果】(1)利用PLS 后,单一
生育期的建模结果较单一植被指数有所改进,但仍有待提高;(2)应用组合预测原理的HI
组合预测模型,显著改善了HI 的估测精度,R2 达到0.55,较单生育期的建模预测,提升了
13%。【结论】基于多生育期信息的组合预测方法,对各单一生育期HI 预测模型赋予最优权
重进行优化组合,实质间接利用了各生育期对作物HI 形成的贡献,显著提高冬小麦收获指
数的估测精度,是一种新颖的作物HI 遥感估测方法。 |
关键词: 收获指数 偏最小二乘法 冬小麦 组合预测法 遥感光谱 多生育期 |
DOI:10.12105/j.issn.1672-0423.20190204 |
分类号: |
基金项目:国家自然科学基金项目(41571416);国家重点研发计划项目(2017YFD0201501) |
|
Estimation of winter harvest index based on PLS andcombined prediction method |
Chen Guo1,2,3, Xu Xingang※2,4, Du Xiaochu1, Yang Guijun2,4, Zhao Xiaoqing2,4, Wei Pengfei1,2,3, Wang Yulong2,4, Fan Lingling2,4
|
1.College of Resources and Environment,Hubei University,Wuhan 430062,China;2.Beijing Agricultural
Information Technology Research Center,Beijing 100097,China;3.National Agricultural Information
Engineering Technology Research Center,Beijing 100097,China );4.National Agricultural Information
Engineering Technology Research Center,Beijing 100097,China
|
Abstract: |
[Purpose]Harvest index can effectively reflect the ability of crop population
photohyalates to be transformed into grain accumulation. Also it is a key index to evaluate the
yield level of crop varieties. Harvest index can be measured in practice. Remote sensing inversion
can save time and manpower,but the accuracy needs to be improved.[ Method]The canopy
spectral data of winter wheat in several key growth stages were used to analyze the correlation
between the selected 44 common vegetation indexes and the measured harvest indexes. Five
optimal typical vegetation indexes are selected in each growth stage. Then,the partial least
squares( PLS) modeling was applied to obtain the HI remote sensing estimation models based on
the spectral information of single growth period. Finally,the combination prediction theory was
used to apply the combination prediction method to assign the optimal weight to each HI spectral
model for each single growth periods,and finally a HI spectral combination prediction model
based on the data of multiple growth periods was constructed.[ Result](1)After using the
PLS,the modeling results of single growth period have improved,compared with that of single
vegetation index. However it still needs to be improved(. 2)The HI combination prediction model
based on the combination prediction principle significantly improved the estimation accuracy of
HI,with R2 up to 0.55,which was 13% higher than the modeling prediction of single growth
period.[ Conclusion]Based on the combination prediction method of information of multiple
growth stages,the optimal weight was given to the HI prediction model of each single growth stage
to optimize the combination. In essence,the contribution of each growth stage to the HI formation
of crops was indirectly utilized to significantly improve the estimation accuracy of winter wheat
harvest index. It is a novel remote sensing estimation method of crop HI. |
Key words: harvest index PLS winter wheat combined forecasting model remote sensing
spectrum growth durations |