本文已被:浏览 6709次 下载 6664次 |
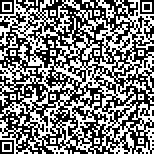 码上扫一扫! |
|
工厂化养殖死鱼检测及定位系统研究 |
赵然1,2,3,4,王宇豪1,2,赵世理1,2,张松1,2,段雨晴1,2
|
1.国家数字渔业创新中心,北京100083;2.中国农业大学信息与电气工程学院,北京100083;3.农业农村部智慧养殖技术重点实验室,北京100083;4.北京市农业 物联网工程技术研究中心,北京100083
|
|
摘要: |
【目的】 在工厂化循环水养殖中,过度密集的养殖环境和不稳定的水质等因素会导致水体污染和鱼类缺氧,进而引发鱼类死亡。因此,及时检测和清除死鱼对于维护水质和防止疾病传播至关重要。【方法】 文章将目标检测模型和双目视觉定位技术相结合,实现对死亡鱼类的实时检测和精准定位。首先采用CLAHE图像增强算法,解决水下死鱼图像面临的光的吸收、散射和折射等问题;其次基于YOLOv7模型构建了死鱼目标检测网络YOLOv7-PC,该网络采用部分卷积模块替换骨干网络中的高效层聚合网络(ELAN)的传统卷积模块,并在Neck网络中引入了坐标注意力机制模块,实现了对被遮挡和水下死鱼目标的实时精准检测;最后结合立体匹配算法SGBM实现对水下死鱼目标的三维定位,为死鱼机器人的打捞提供了视觉感知信息。该研究以大口黑鲈为例,基于不同距离采集的视频数据集进行测试,验证了YOLOv7-PC模型结合SGBM立体匹配算法的准确性和实时性。【结果】 该研究提出的YOLOv7-PC-CLAHE模型精确率、召回率及平均精度分别为97.6%、85.6%和97.0%,对比基础YOLOv7模型平均精度提高了2.5%,检测速度提升了31.9%;结合SGBM立体匹配算法能够精准进行死鱼定位,在深度方向上平均相对误差为3.08%。【结论】 该文方法有效实现了对水下死亡鱼类的实时检测和精准定位,算法效果满足工厂化养殖的需求。 |
关键词: 图像增强 死亡鱼类检测 YOLOv7 双目立体视觉 立体匹配 三维定位 |
DOI:10.12105/j.issn.1672-0423.20240203 |
分类号: |
基金项目:国家自然科学基金项目“基于计算机视觉和多维数据时空耦合的游泳性鱼类患病程度表征模型研究”(32273188) |
|
Detection and positioning system of dead fish in factory farming |
Zhao Ran1,2,3,4, Wang Yuhao1,2, Zhao Shili1,2, Zhang Song1,2, Duan Yuqing1,2
|
1.National Innovation Center for Digital Fishery,Beijing 100083,China;2.College of Information Electrical Engineering,China Agricultural University,Beijing 100083,China;3.Key Laboratory of Smart Farming Technologies for Aquatic Animal and Livestock,Ministry of Agriculture and Rural Affairs,Beijing 100083,China;4.Beijing Engineering and Technology Research Center for Internet of Things in Agriculture,Beijing 100083,China
|
Abstract: |
Purpose In factory-based circulating water breeding,excessively dense breeding environment and unstable water quality have caused water pollution and hypoxia,resulting in fish mortality. Therefore,timely detection and removal of dead fish is essential for maintaining water quality and preventing diseases from spreading.Method This article proposed a combination of the target detection model and the visual positioning technology of the eyes to achieve real-time detection and accurate positioning of dead fish. Firstly,the CLAHE image enhancement algorithm was used to solve the problems such as the absorption,scattering and refraction of the light facing the underwater dead fish images. Secondly,the YOLOv7-PC was built based on the YOLOv7 model. The traditional convolutional module of the high-efficiency layer aggregation network(ELAN)was replaced by a Partial Convolution (PConv) module, and a coordinate attention mechanism module was introduced into the Neck network to achieve real-time accurate detection of obscured and underwater dead fish targets. Finally, combined with the Semi-Global Block Matching (SGBM) to achieve three-dimensional positioning of underwater dead fish targets, which provided visual perception information for the salvage of dead fish robots. Taking the large-mouth black bass as an example, the accuracy and real-time performance of the YOLOv7-PC model combined with the SGBM stereo algorithm was verified by testing the video data collected at different distances.Result The test results showed that the accuracy,recall rate and average accuracy of the Yolov7-PC-CLAHE model proposed in the study were 97.6%,85.6% and 97.0%,respectively. The average accuracy of the comparison base YOLOv7 model had increased by 2.5% and the detection speed had increased by 31.9%. Combined with the SGBM three-dimensional algorithm algorithm could accurately perform dead fish positioning,the average relative error in the depth direction was 3.08%.Conclusion The method proposed in this article effectively realizes real-time detection and accurate positioning of underwater dead fish,and the algorithm's performance meets the needs of industrialized aquaculture. |
Key words: image enhancement death fish detection YOLOv7 two-dimensional vision three-dimensional matching three-dimensional positioning |