引用本文: | 李中元,郑正彬,林博文,唐锰,蔡祎晨,王新生,汪权方,陈志杰.黄桃种植区提取及其潜在适生区预测[J].中国农业资源与区划,2022,43(5):74~83 |
| |
|
|
|
本文已被:浏览 547次 下载 487次 |
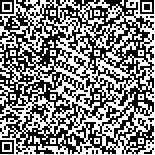 码上扫一扫! |
|
黄桃种植区提取及其潜在适生区预测 |
李中元1,2,3,郑正彬1,2,3,林博文1,2,3,唐锰1,2,3,蔡祎晨1,2,3,王新生1,2,3,汪权方1,2,3,陈志杰1,2,3
|
1.湖北大学资源环境学院,武汉 430062;2.区域开发与环境响应湖北省重点实验室,湖北武汉 430062;3.湖北省农业遥感应用工程技术研究中心,武汉 430062
|
|
摘要: |
目的 产业振兴是乡村振兴的一项重要内容,发展乡村产业,对我国乡村振兴战略的实施有重要意义。黄桃是湖北省江陵县三湖农场的特色农产品,通过识别黄桃种植区并预测其潜在适生区,将有助于黄桃生产空间布局优化。方法 文章选取哨兵二号遥感影像,利用时间序列植被指数、物候特征以及随机森林分类器方法对研究区黄桃种植区进行提取。在此基础上,选取环境变量,使用最大熵模型MaxEnt对黄桃在江汉平原的潜在适生区进行预测。结果 (1)黄桃开花是利用遥感进行黄桃种植区提取的重要物候特征,黄桃种植区提取的总体精度为87%,kappa系数为0.84,分类精度较好,可满足应用要求;(2)最大熵模型MaxEnt精度显示AUC值为0.993,说明预测结果可靠,可用于黄桃潜在适生区的预测;(3)黄桃的潜在适生区主要位于江陵县中北部、沙市区南部、潜江市东南部以及公安县东北部,宜城市和京山县北部也适宜黄桃的种植;(4)最高及最低太阳辐射、最低气温、高程为影响黄桃生长的主要环境变量。结论 该研究成果能够为黄桃的空间布局优化与乡村振兴提供理论指导和决策依据。 |
关键词: 哨兵二号 物候 遥感 黄桃 随机森林 MaxEnt |
DOI:10.7621/cjarrp.1005-9121.20220508 |
分类号:P237 |
基金项目:湖北省技术创新专项重大项目“基于天空地协同观测的精准农情监测技术研发与示范”(2018ABA078);中国科学院A类战略性先导科技专项资助“扶贫富民路径提升关键技术与示范”(XDA23070400) |
|
EXTRACTION OF PLANTING AREA AND PREDICTION OF POTENTIAL SUITABLE DISTRIBUTION OF YELLOW PEACH |
Li Zhongyuan1,2,3, Zheng Zhengbin1,2,3, Lin Bowen1,2,3, Tang Meng1,2,3, Cai Yichen1,2,3, Wang Xinsheng1,2,3, Wang Quanfang1,2,3, Chen Zhijie1,2,3
|
1.Faculty of Resources and Environment Science, Hubei University, Wuhan 430062, Hubei, China;2.Hubei Key Laboratory of Regional Development and Environmental Response, Wuhan 430062, Hubei, China;3.Hubei Engineering Research Center for Remote Sensing Technology in Agriculture, Wuhan 430062, Hubei, China
|
Abstract: |
Industry revitalization is one of the important contents of rural revitalization. It is of great significance to the implementation of China's rural revitalization strategy for developing rural industries. Yellow peach (Amygdalus persica L.) is one of the distinctive agricultural products in Sanhu Farm, which is located in Northeast Jiangling county of Hubei province. Accurately predicting suitable cultivation areas of Yellow peach from satellite observations can be useful for optimization spatial distribution of planting area. In this study, an approach based on Sentinel-2 satellite data and the MaxEnt model was developed for predicting suitable cultivation areas of Yellow peach. Time series vegetation indices (e.g., NDVI, NDWI and RVI) derived from the Sentinel-2 satellite data were first used to identify the planting area of Yellow peach in 2019. Specifically, phenological metrics (e.g., date of the flowering stage) were extracted from the vegetation indices and were inputted of a Random Forest (RF) classifier for planting area classification. And the MaxEnt model was calibrated to obtain relationships between environmental factors (e.g., terrain, vegetation, meteorological and soil) and planting area for predicting suitable cultivation areas. The results were showed as follows. (1) The flowering stage derived from satellite data had the most contribution on identification the plating area of the Yellow peach. The overall accuracy of the best classification model was 87%, and the kappa coefficient was 0.84. (2) The mean value of the AUC (Area Under Curve) indicator for the calibrated MaxEnt model was 0.993, indicating the calibrated model was reliable for predicting the potentially suitable cultivation areas of Yellow peach. (3) Prediction showed that the potentially suitable cultivation areas mainly distributed in central Jianghan Plain, such as north-central of Jiangling county, south of Shashi district, southeast of Qianjiang city and northeast of Gong'an county. Other cultivation areas, such as the north Yicheng and Jingshan county were good for planting as well. (4) The research also showed that the distribution of the planting area of Yellow peach was mainly affected by environmental factors including daily maximum and minimum solar radiation, daily minimum air temperature, and elevation. This research can provide theoretical suggestions for obtaining optimization in the planting area of Yellow peach and rural revitalization. |
Key words: Sentinel-2 phenology remote sensing Amygdalus persica L. random forest MaxEnt |
|
|
|
|
|
|