引用本文: | 孙小香,王芳东,赵小敏,谢文,郭熙.基于冠层光谱和BP神经网络的水稻叶片氮素浓度估算模型[J].中国农业资源与区划,2019,40(3):35~44 |
| |
|
|
|
本文已被:浏览 1196次 下载 433次 |
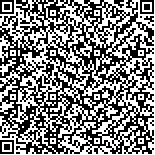 码上扫一扫! |
|
基于冠层光谱和BP神经网络的水稻叶片氮素浓度估算模型 |
孙小香, 王芳东, 赵小敏, 谢文, 郭熙
|
江西农业大学江西省鄱阳湖流域农业资源与生态重点实验室/南方粮油作物协同创新中心,南昌330045
|
|
摘要: |
[目的]快速、准确地诊断水稻叶片氮素营养状况,为水稻氮肥精准管理提供依据。[方法]以江西省农科院8种不同施肥处理的晚稻为研究对象,于主要生育期同步测定了水稻冠层光谱反射率及叶片全氮浓度(Leaf Nitrogen Concentration,LNC),系统分析了原始光谱反射率、一阶微分光谱、“三边”参数以及由350~1 350nm两两波段组合的差值(SD(Rλ1,Rλ2))、比值(SR(Rλ1,Rλ2))及归一化(ND(Rλ1,Rλ2))光谱指数与水稻LNC的相关关系,筛选出敏感参数,并以之为自变量构建了水稻LNC的传统预测模型,另外构建不同指标个数的多元线性与BP神经网络模型,并对模型进行验证。[结果](1)水稻LNC与一阶微分光谱在751nm处的相关性最高(r=0822); (2)“三边”参数中的红边面积SDr与LNC的相关性较高(r=0687); (3)750nm附近的红边波段与近红外波段差值组合、550nm附近的绿光波段与近红外波段的比值及归一化差值组合与水稻LNC的相关性较高,以SD(R752,R751)、SR(R534,R1 350)和ND(R534,R1 349)表现最好,相关系数分别为0827、-0790和0788; (4)传统回归模型中以SD(R752,R751)构建的一元线性模型最佳(RC2=0665、RV2=0750、RMSEV=04%、RPD=2034); (5)利用5个指标((R′751、SDr、SD(R752,R751)、SR(R534,R1 350)、ND(R534,R1 349))经逐步回归筛选出的2个指标SD((R752,R751)和SR(R534,R1 350))构建预测水稻氮素的BP神经网络模型,预测效果最佳,其验证参数值分别为R2=0859、RMSEV=0302%和RPD=2669。[结论]基于单指标构建的传统线性模型计算过程简单但精度略低,而基于2个指标(SD(R752,R751)、SR(R534,R1 350))构建的BP神经网络模型预测精度高于该2指标构建的多元线性模型,表明在指标适合的情况下,BP神经网络对氮素具有较好的预测能力,是一种快速准确估算水稻叶片全氮浓度的方法。 |
关键词: 水稻高光谱冠层叶片全氮浓度BP神经网络 |
DOI: |
分类号:S127 |
基金项目:国家自然科学基金(41361049)国家重点研发计划资助(2017YFD0301603);江西省赣鄱英才“555”领军人才项目(201295) |
|
THE ESTIMATION MODELS OF RICE LEAF NITROGEN CONCENTRATION BASED ON CANOPY SPECTRUM AND BP NEURAL NETWORK |
Sun Xiaoxiang, Wang Fangdong, Zhao Xiaomin, Xie Wen, Guo Xi
|
Key Laboratory of Poyang Lake Watershed Agricultural Resources and Ecology of Jiangxi Province, Jiangxi Agricultural University/Southern Regional Collaborative Innovation Center for Grain and Oil Crops China , Nanchang, Jiangxi 330045, China
|
Abstract: |
The objective of the study is to analyze the relationships between canopy spectral reflectance characteristics and leaf nitrogen concentration(LNC), and establish the rapid and accurate method to diagnose the rice leaf nitrogen nutrition by spectrum, so as to provide the basis of rice precision fertilization. An experiment of eight different fertilization treatments on the late rice was carried out in the Jiangxi Academy of Agricultural Sciences. The canopy spectral reflectance and leaf nitrogen concentration(LNC) were measured simultaneously in the main growth stages which were stages of tillering, booting, heading, maturity. The original spectral reflectance, the first derivative spectrum, the 'three edge' parameter and the spectral index including difference(SD (Rλ1, Rλ2)), the ratio(SR (Rλ1, Rλ2)) and the normalized(ND (Rλ1, Rλ2)) between 2 bands from spectral range in 350 1350nm and their relationship with rice LNC were systematically analyzed to selected the sensitive parameters, and constructed the traditional prediction model of rice LNC with the sensitive parameters as the independent variables. In addition, the multiple linear model and BP neural network model of different index number were established, and the models were verified. The results were showed as follows. Firstly, the maximum correlation coefficient was between the rice LNC and the first order differential spectrum at 751nm (r=0.822). Secondly, the correlation coefficient between the "red edge"area(SDr) in the "three edge" parameters and the LNC was high(0.687). Thirdly, the correlation coefficients between the rice LNC and the difference spectral index of red edge band near 750 nm with Infrared band, between the rice LNC and the ratio of green band near 550nm with Infrared band, and between the rice LNC and the normalized difference spectral index were high, with SD (R752, R751), SR (R534, R1 350), and ND (R534, R1 349) were best, and the correlation coefficients were 0.872, -0.790 and 0.788, respectively. Fourthly, in the traditional regression models, the linear model of SD (752, 751) was the best (Rc2=0.665, Rv2=0.750, RMSE=0.4%, RPD=2.034). Finally, using 2 indicators((R752, R751), SR(R534,R1 350)), which were selected from 5 indicators ((R′751, SDr, SD (R752, R751), SR (R534, R1 350), ND (R534, R1 349)) through stepwise regression, a BP neural network model to predict rice nitrogen was built. The model had the best prediction effect, with the accuracy of the model were R2=0.859, RMSEv=0.302% and RPD=2.669, respectively. Comparing with the single index traditional regression models, mult index models has higher accuracy, especially two index BP neural network model which has the highest accuracy. The result show that BP neural network model is a quick and accurate method to estimate the rice LNC in the case of suitable index. |
Key words: rice hyperspectral canopy leaf nitrogen concentration BP neural network |
|
|
|
|
|
|